As the reality sinks in that the shift to online education will continue indefinitely—and some of it permanently—now is a particularly good time to re-examine our beliefs about effective teaching.
We know from research and experience that many educators who have made the transition to online teaching in the past have rethought their teaching practices. I have been through this myself. Some techniques that always worked for me in a face-to-face classroom failed utterly online. Anything that relies on personal charisma—especially when trying to provoke discussion—can easily fall flat. Other techniques worked only after I stripped them down to first principles and translated them into the new medium. Still others that seemed like they would never work in a face-to-face setting did in a virtual one.
When our teaching practice is rooted in instinct, tradition and occasional trial-and-error experiments, we often bundle together different aspects of what we are doing without ever teasing out which teaching moves are the critical ones—and why. A radical switch to a new teaching environment, where many contextual factors change dramatically, causes many first-time online teachers to take a hard look at their assumptions. They may learn, for example, that the Q&A portion of their lecture class was actually the part where students learned the most, rather than from the meticulously crafted presentation from the lectern.
The problem is that many educators, having accomplished the hard introspection needed to teach well online, stop there. They don’t develop a practice of introspection and methodical experimentation to continuously improve. While they may have learned to be better teachers during their transition to online, they often do not learn how to be better learners.
One reason may be that nobody has told them that this is something they can or should be doing. Having been professionalized into a world of disciplinarity, where the subject matter they research and teach is the center of their professional life, nobody has told them that there is a discipline for continuous improvement of teaching, never mind that they could be expert practitioners of this discipline.
But there is such a discipline focused on teaching practice, and it’s called learning engineering.
Double-Loop Learning
Good educators are empirical in the sense that they are constantly testing to see what their students know, what they are missing, which concepts they appear to be stuck on, which lessons ignite their passion and curiosity, which activities cause them to get bored and drift, and so on. Educators create feedback loops that enable them to continuously adjust their teaching to meet the needs of the students who are in front of them.
Most faculty, having never been formally educated on how to be an educator, do this by instinct. Some learn the craft from their colleagues, or from books, or professional support staff or other sources. Under ordinary circumstances, many of the better online education programs require instructors to take formal training before they teach their first online course. These courses usually teach faculty how to create good feedback loops in an online environment. Many also teach evidence-based principles of good teaching. Faculty can develop novel ideas for feedback loops by applying the principles in new ways.
For example, educators who routinely but informally quiz their students during class discussions learn to name what they are doing as “formative assessment.” Having named the instinct and turned it into a tool that they can pick up at will, they are able to think more creatively about opportunities to make use of formative assessments in an online context.
But very few of these training programs teach faculty how to test and interrogate the principles themselves. At this moment of opportunity when many educators are not only open to but eager for help in rethinking their practice, we miss the opportunity to teach them that evidence-based principles of effective teaching are still just hypotheses, that these hypotheses can be tested in the classroom, and that instructors are often in an excellent position to test and refine them, improving both their personal teaching practice and the state of the art in the profession.
Learning engineering starts with the recognition that our ideas about effective teaching practices are based on what we think we know about how students learn. And we might be wrong about what we think we know. When we test what we think will work, we should also test our theory as to why we think it will work.
This is how the Simon Initiative at Carnegie Mellon University—the birthplace of learning engineering—illustrates the concept:
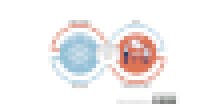
The circle in the center, labeled “model of expertise,” is the educator’s theory of what it means to develop competence in the discipline being taught. It’s our idea of what it means to think like, say, a mathematician, or a sociologist or a philosopher. The loop on the right is the traditional feedback loop. We design our courses to teach students how to become experts, we see how students respond and we adjust our design accordingly.
The loop on the left is the new one. It tells us what to do if students don’t respond as we expect them to when we adjust our teaching based on the traditional teaching feedback loop. Students get stuck, and the adjustments we make to help them don’t get them unstuck. This happens in the classroom all the time. But because educators are not given a framework in which to think about it, they don’t learn from these experiences as often as they could. And even when they do learn, they often lack the vocabulary to share what they have learned with their colleagues.
Data and Models
The two words in the graphic above that may put off some faculty—depending on which discipline they are in—are “data” and “model.” Of these, “data” is the one that seems to make educators in some disciplines more uncomfortable.
It shouldn’t.
In every discipline, we learn by abstracting the object of study to remove details that don’t matter. For example, we analyze a novel or a poem by focusing on elements that show the author’s pattern of meaning while ignoring those elements that don’t. Sometimes we do a better job in this abstraction than others, but we are always looking for patterns by foregrounding some pieces while relegating others to the background. In the context of learning engineering, “data” should be thought of as a means by which educators can find patterns of meaning through abstraction. The fact that the elements we are choosing to analyze are digital artifacts is largely incidental to the thought process. A student who doesn’t log into their learning environment is a student who doesn’t show up for class. I don’t mean to trivialize either the affordances or the complications that come from digitizing these abstractions. My point is simply that “data” aren’t meaningful in a learning engineering context unless they are meaningful to the educator. They have to make sense in a way that enables us to examine and reconsider our theories and teaching practice.
The more difficult word in the Carnegie Mellon diagram is actually “model.” What does it mean to have a “model of expertise”?
Most educators have some internalized idea of how they will know if their students are becoming experts in the subject being taught, as well as what is required to help them achieve that expertise. But relatively few professors have been required to explicitly articulate their ideas in ways that they can test.
This is the heart of learning engineering. Educators need to be clear about what skills they are teaching, and how students learn those skills. How does a student learn to interpret a poem? Solve a linear equation? Determine a company’s valuation? If we can’t provide testable and falsifiable answers to these questions, then we have a sharp limit on how much we can improve our teaching. Specifically, we can only improve our teaching to the degree that our initial ideas about how students learn to become experts are correct.
At a moment when many of our assumptions about learning are being put to the test as many of the teaching conditions that we used to be able to assume are changing during the pandemic, now seems like a particularly good time to cultivate the discipline of learning engineering among all educators.